Optimal scheduling of electricity-gas-heat-hydrogen integrated energy system considering carbon transaction cost
Abstract
Aim: To improve energy efficiency, optimize the operation of equipment, and reduce the carbon emission of the integrated energy system (IES), an IES low-carbon operation strategy is proposed.
Methods: This paper comprehensively considers the influence of the stepped carbon trading scheme, the wind generation and load forecasting represented by fuzzy parameters, and the operation strategy of energy storage equipment with flexible space margin on the optimal scheduling of IES. Based on this, a low-carbon operation target with the minimum sum of power purchase cost, gas purchase cost, carbon emission cost, and abandoned wind cost is constructed.
Results: Five scenarios are compared and analyzed. The effects of different carbon emission penalty strategies and wind power output and load uncertainties on benefits are analyzed. The feasibility of the proposed scheme is verified by the simulation results of a numerical example.
Conclusion: The stepwise carbon trading mechanism can better guide the effect of carbon emission reduction compared with the traditional carbon trading pricing model.
Keywords
INTRODUCTION
With the integration of large-scale renewable energy sources, their randomness and intermittence have led to the problem of wind and light abandonment[1,2]. The integrated energy system (IES) of microgrids supports access of large-scale renewable energy, a variety of energy conversion and storage equipment[3,4]. Its energy complementarity has the functions of flexible consumption of renewable energy and reduction of carbon emissions, which provides ideas for low-carbon scheduling of the system. The operation optimization of electricity-gas-heat-hydrogen IES has been a research hotspot in this field[5,6].
At present, the research on IES focuses on economic dispatch. Ref[7] considered the role of power-to-gas (P2G) equipment in the electricity-gas-heat IES and its contribution to improving the system economy. An economic dispatch model of cogeneration units with P2G and thermal electrolysis coupling is proposed in ref[8]. This model can improve the economy through the coordinated operation of P2G and cogeneration. In the actual production process, P2G technology is usually divided into two processes: electrochemical hydrogen production and methanation; that is, hydrogen is first produced by electrolysis, and then hydrogen and carbon dioxide are synthesized into methane. After multiple energy conversions, the efficiency of the power and gas energy closed-loop is less than 50%, and the investment cost is increased. To seek a more efficient operation mode[9,10]. Ref[11] combines wind power hydrogen production with local hydrogen storage tanks and fuel cells to participate in a day-ahead economic dispatch and verifies that the use of power-to-hydrogen technology brings better environmental protection and economy to the system but does not consider the use of gas network hydrogen blending technology and gas storage to further reduce energy storage costs. The uncertainty of wind generation and load forecasting brings great challenges. For the treatment of prediction error, the ref[12] found that it obeys Beta distribution, but the error is too idealized according to a specific probability distribution. In ref[13], the constraints with fuzzy parameters are expressed as fuzzy chance constraints, but the fuzziness of load is not considered. Ref[14] pointed out that flexibility is the inherent characteristic of a power system and has the characteristics of direction and time scale. The ref[15] further points out that flexibility has the characteristics of supply diversity and demand uncertainty; different from power supply reliability, operational flexibility reflects the ability to maintain active power balance under continuous uncertain events[16]. The complementarity of IES energy flows in the transmission process can improve the operational flexibility[17]. In the electrical interconnection system model considering natural gas storage, the use of gas turbines with fast start-stop can improve the operational flexibility[18]. Ref[19] shows that multi-energy coupling equipment can use the difference of energy transmission characteristics of each network to achieve coordinated operation so as to improve the operational flexibility.
In the context of increasingly prominent environmental problems, reducing carbon emissions has become an important indicator for measuring IES. Carbon trading is an effective measure to reduce carbon emissions[20,21]. In[22], A non-power conversion strategy is proposed and implemented to compare the charging and discharging priorities of hydrogen and batteries. In[23-25], various methods, such as deep reinforcement learning, data-driven techniques, and Newton-Raphson algorithms, are used to study the optimal scheduling problem of IESs. Ref[26] proposed an optimal scheduling model with a carbon trading mechanism and analyzed the impact of carbon trading on the economy and carbon emissions, which has guiding significance for the economic scheduling of IES. At the same time, a few references introduce carbon trading mechanisms into the source-side centralized scheduling of electricity-gas-heat-hydrogen IES. The existing research often requires the energy storage equipment to balance the replay power during the daily scheduling period to ensure that the capacity state of the energy storage equipment remains unchanged at the beginning and end of the day. A reasonable flexibility margin should be allowed to improve the utilization efficiency of energy storage equipment. In addition, few pieces of literature comprehensively consider the impact of stepped carbon trading mechanism, detailed P2G two-stage operation, and collaborative operation with high output and load uncertainty on IES scheduling.
Therefore, based on the above research, this paper comprehensively considers the influence of the stepped carbon trading mechanism, the wind generation and load forecasting represented by fuzzy parameters, and the operation strategy of energy storage equipment with flexible space margin on the optimal scheduling of IES and constructs the operation target with the minimum total cost. The problem is transformed into a mixed integer linear problem, which is solved by a CPLEX commercial solver.
EQUIPMENT MODEL OF IES
IES includes the energy supply, conversion, storage equipment, and load, which can realize the coordinated optimization and complementary operation of various forms of energy. The IES structure with electricity-gas-heat-hydrogen is shown in Figure 1.
Figure 1. The IES structure with electricity-gas-heat-hydrogen. CHP: Combined heat and power generation; EL: electrolyze; GB: gas boiler; HFC: hydrogen fuel cell; MR: methane reactor.
Due to the anti-peak regulation characteristics of wind generation, it is necessary to be equipped with corresponding energy conversion and storage equipment. P2G technology is an important means to absorb wind curtailment, and it can be divided into two parts: Electrolyze (EL) and Methane Reactor (MR). EL converts electricity into hydrogen, while MR further converts it into gas. Additionally, it can also be directly fed to the hydrogen fuel cell (HFC) to realize the conversion of hydrogen to electricity and thermal energy. A gas boiler (GB) burns gas and provides heat. Combined heat and power generation (CHP) produces electricity and heat simultaneously by burning gas. In addition, IES includes electric, gas, heat, and hydrogen energy storage to achieve the optimal economic benefit of integrated energy.
CHP
CHP generates electricity by burning gas and supplies the heat load with the waste heat generated during the power generation process. The CHP, with an adjustable heat-to-electric ratio, can adjust the output of electricity and heat according to the real-time demand of electricity and heat energy and further optimize operational efficiency. Its working model is:
Where
EL
EL first converts electricity into hydrogen. Part of the hydrogen is input into MR and CO2 to synthesize gas, which is supplied to the gas load, GB, and CHP; part is directly transmitted to HFC to convert electricity and thermal energy, and part is stored by the hydrogen storage tank. Hydrogen is directly converted into electrical and heat through HFC. Compared with the first conversion to gas and then combustion supply through GB or CHP, it reduces an energy conversion link and reduces the cascade loss of energy. The energy efficiency of hydrogen is higher than that of gas, and no CO2 is generated. The direct supply of hydrogen to HFC has more benefits. The above conversion model can be described as:
Where
MR
The energy conversion model of MR can be described as:
Where
HFC
Ref[27] shows that the sum of the thermal and electrical energy conversion efficiency of HFC can be regarded as a constant, and the electrical and thermal conversion efficiency can be adjusted. Therefore, the HFC model with an adjustable thermoelectric ratio can be constructed:
Where
GB
The energy conversion model of GB can be described as:
Where
Wind generation constraints
Where Pw(t) is the wind generation, and
THE OPTIMAL SCHEDULING MODEL
Objective function
The economic optimization operation of the microgrid IES requires the minimum total operating cost. Wind turbines use natural resources to generate electricity and can be considered to have no power generation cost. Therefore, this paper mainly considers the electricity/gas purchase cost
Considering that the wind power in the microgrid with wind power has great randomness and volatility, the power quality cannot meet the requirements of power transmission to the grid. To reduce the pressure of the main network, this paper only considers the one-way power supply from the grid to the microgrid. The cost of electricity purchase and gas purchase is:
Where
The wind curtailment cost is:
Where cw is the unit wind curtailment cost, and Pw,out(t) is the wind curtailment power.
The regulatory authorities first allocate carbon emission quotas for each carbon emission source and its producers. If the carbon emission is less than the free carbon quota, the manufacturer can sell the excess carbon emission quota and obtain a part of the incentive subsidy; otherwise, it needs to purchase the insufficient emission rights. The greater the carbon emissions, the higher the trading price.
Carbon emission quota
The carbon emission sources in IES are GB, CHP, and superior power purchase. At present, the superior power purchase comes from coal-fired units.
Where EIES and
Actual carbon emission
The hydrogen-to-gas conversion of MR can absorb CO2. The actual carbon emission model is as follows:
Where EIES.act and
Step carbon emission trading
The carbon emission trading volume participating in the carbon trading market is:
Where, EIES.trade is the carbon emissions trading volume.
This paper adopts a stepwise pricing mechanism. The stepped carbon transaction costs are:
Where λ is the base price of carbon trading, l is the length of carbon emission interval, and α is the price growth rate. In this paper, l = 2t, α = 25%, and λ = 250 ¥/t. The stepped pricing mechanism divides several purchase intervals, and the higher the purchase price of the corresponding interval, the more carbon emission permits need to be purchased.
Constraint condition
Equipment constraints
As shown in Equations (1)-(6).
Energy storage constraints
According to the ref[28], the models of energy storage, such as electricity, heat, and gas, are similar, so this paper models the electricity, heat, gas, and hydrogen energy storage equipment uniformly. In the current research and practice of optimal scheduling of energy storage equipment, it is often required to balance the charging and discharging power in the daily scheduling cycle to ensure that the capacity state is unchanged at the beginning and end of the day. But in fact, a reasonable flexibility margin should be allowed at the first and last moments. If the release capacity demand of the system for energy storage equipment in one day is greater than the storage capacity demand, the capacity at the end time should be allowed to be slightly lower than the initial time. Similarly, when the demand of the system for storage capacity is greater than the release capacity demand in one day, the end-time capacity should be allowed to be slightly higher than the initial moment. SES(1) is the capacity state of the energy storage at the initial time, and the water level at the end time can be flexibly changed in the interval SES(1) ± kESSES.C; it is the flexible margin space. Among them, SES.C is the rated capacity, and kES is the flexible margin coefficient. Therefore, based on the optimal scheduling model of conventional energy storage equipment, this paper considers the flexible margin space constraint to improve the capacity utilization efficiency. The constraints of various types of energy storage equipment are:
Where the superscript “type” represents the energy storage type.
Purchase constraints of electricity and gas
Where
Power balance constraint
The power balance equations are:
Where
FUZZY CHANCE CONSTRAINTS
Due to the uncertainty of wind generation, electric, heat, and gas load, some constraints in the model should be specially treated. Fuzzy chance-constrained programming is used to describe the uncertainty.
The original problem is:
Where f(x) is the objective function, ξ is the fuzzy variable vector, Cr·
Trapezoidal fuzzy parameters are used to represent fuzzy variables in this paper. The membership function of trapezoidal fuzzy variables is:
Where r1 < r2 ≤ r3 < r4, if the predicted output or load is r0, then:
Where ωi is the proportional coefficient, and its value can be determined according to historical data.
The key to solving the fuzzy chance-constrained programming problem is to conduct the chance constraints. In this paper, the uncertain constraints containing fuzzy variables are transformed into deterministic constraints to solve the model. In[29], the principle and transformation method of a clear equivalence class is introduced in detail. Therefore, this paper directly deals with the clear equivalence class of Equations (16)-(18). In the most unfavorable case, the power balance equations are transformed into:
Where αe, αh and αg are the confidences satisfying the constraints.
EXAMPLE ANALYSIS
This paper proposes a mixed integer linear programming (MILP) problem for the optimal scheduling model of electricity-gas-heat-hydrogen microgrid considering carbon transaction costs, and CPLEX is used to solve the large-scale MILP.
Considering that there are nonlinear expressions in the IES model, such as the square term of thermal power output in Equation (11), it is difficult to solve it directly, and the solution time is long. Therefore, this paper uses the incremental linearization method with quadratic constraints to linearize it respectively.
The prediction results of various loads and wind generation are shown in Figure 2. The electricity price and gas price are shown in Figure 3. The carbon emission quota parameter is 0.798 kg/(kW·h), the carbon emission quota parameter is 0.385 kg/(kW·h), and the wind abandonment penalty cost parameter is
Three operating scenarios are set up for analysis. Scenario 1 is a traditional economic dispatch scenario in which the optimization goal does not consider carbon transaction costs but only considers energy purchase costs and wind curtailment costs. Scenario 2 is an economic dispatch scenario under the traditional carbon trading mechanism. Scenario 3 is an economic dispatch scenario under the stepped carbon trading mechanism. Table 1 shows the scheduling results under three operating scenarios, and the output of each device is shown in Figures 4-6. According to the table, the carbon emissions when the optimization goal considers the carbon transaction cost is much smaller than the optimization goal without considering the carbon transaction cost. Among them, Scenario 3 has the least carbon emissions. Considering the stepped carbon trading mechanism can achieve the purpose of emission reduction. The microgrid in the example is characterized by small wind power and large thermal and electrical loads. Due to the high cost of additional power purchase and gas purchase, the optimization algorithm tends to give priority to the use of wind power, so there is no curtailment cost in the calculation results of each scenario.
Figure 4. The output of each device in Scenario 1. (A) electric power; (B) heat power; (C) hydrogen power; (D) gas power.
Figure 5. The output of each device in Scenario 2. (A) electric power; (B) heat power; (C) hydrogen power; (D) gas power.
Figure 6. The output of each device in Scenario 3. (A) electric power; (B) heat power; (C) hydrogen power; (D) gas power.
The scheduling results under Scenarios 1-3
Scenario | Actual carbon emissions/kg | Carbon emission quota/kg | Carbon emission trading/kg | Carbon trading cost/¥ | Electricity purchase cost/¥ | Gas purchase cost/¥ | Wind curtailment cost/¥ | Total cost/¥ |
1 | 20,507 | 8,563 | 11,944 | 4,722 | 158 | 9,129 | 0 | 14,009 |
2 | 18,218 | 9,031 | 9,187 | 2,298 | 1,023 | 8,633 | 0 | 11,954 |
3 | 16,502 | 9,715 | 6,787 | 2,219 | 2,220 | 8,199 | 0 | 12,638 |
According to the electricity and gas prices, Scenario 1 aims at the traditional economic operation optimization. Because the gas price is lower than the electricity price in each period, the system will buy as much gas as possible and supply to the electric load through CHP, so the total energy purchase cost is the smallest. However, the actual carbon emissions generated by burning gas due to the large purchase of gas are much higher than the carbon emission permit quota. Many carbon emission permit quotas need to be purchased from the trading market, so the total cost is the largest; in Scenario 2, the carbon transaction cost is considered. Although the purchase of gas is cheaper than the purchase of electricity, because the system is burning gas in a high carbon emission state at this time, the cost of purchasing electricity instead of gas is lower than the cost of purchasing carbon emission quotas from the carbon trading market due to the high carbon emissions generated by burning gas. Therefore, compared to Scenario 1, Scenario 2 reduces the purchase of gas and increases the purchase of electricity; in Scenario 3, the purchase price of carbon emission quotas increases step by step due to the ladder-type carbon trading mechanism, which further limits the carbon emissions of the system to a certain extent. Therefore, Scenario 3 reduces gas purchases again, increases electricity purchases, and reaches a new balance. Although the total cost of Scenario 3 is 684 yuan higher than that of Scenario 2, the carbon emission is reduced by 1,716 kg, which shows that the system can reduce emissions while ensuring lower operating costs.
The uncertainty of wind generation and electric, heat, and gas loads will have an impact on the optimal operation economy of the microgrid. Based on Scenario 3, the influence of uncertainty of wind generation and load on economic benefits is analyzed. If the confidence level is fixed at 0.9, the trapezoidal fuzzy membership parameters of wind generation are shown in Table 2. Under the ambiguity degree 1, the wind generation and load are both determined values (Scenario 3). Under the ambiguity degree 2, only the uncertainty of wind generation (Scenario 4) and the wind generation uncertainty and load (Scenario 5) are considered. The output of each device is shown in Figures 7 and 8, and the conclusion results are shown in Table 3.
Figure 7. The output of each device in Scenario 4. (A) electric power; (B) heat power; (C) hydrogen power; (D) gas power.
Figure 8. The output of each device in Scenario 5. (A) electric power; (B) heat power; (C) hydrogen power; (D) gas power.
The trapezoidal fuzzy membership parameters
Fuzzy degree | ω1 | ω2 | ω3 | ω4 |
Degree 1 | 1.00 | 1.00 | 1.00 | 1.00 |
Degree 2 | 0.90 | 0.95 | 1.05 | 1.10 |
The scheduling results under Scenarios 4-5
Scenario | Actual carbon emissions/kg | Carbon emission quota/kg | Carbon emission trading/kg | Carbon trading cost/¥ | Electricity purchase cost/¥ | Gas purchase cost/¥ | Wind curtailment cost/¥ | Total cost/¥ |
4 | 21,933 | 12,409 | 9,524 | 3,512 | 3,718 | 9,054 | 0 | 16,284 |
5 | 27,980 | 14,068 | 13,912 | 5,706 | 4,300 | 10,136 | 0 | 20,142 |
When only taking the wind generation uncertainty into account (the actual output is less than the predicted value), the power purchase cost of the system under the fuzzy degree 2 is 3,718 ¥, the gas purchase cost is 9,054 ¥, and the total cost is 16,284 ¥. According to Figure 7, there are more power purchase behaviors in the whole period. This is because the wind generation is uncertain, and the system needs to purchase more electricity and gas as backup power. When taking the wind generation uncertainty and load into account, the power purchase cost of the system is 4,300 ¥, the gas purchase cost is 10,136 ¥, and the total cost is 20,142 ¥. By comparing the results shown in Figures 7 and 8, when considering the load uncertainty (the actual load is greater than the predicted value), to meet the load energy supply, the cost of electricity and gas purchase is further increased.
The confidence level reflects the ability of decision-makers to control risks. In the scheduling model of this paper, the risk comes from the wind generation uncertainty and load forecasting, which makes it difficult to meet the system power balance equation constraints. Therefore, actual decision-making can be introduced.
CONCLUSIONS
In this paper, the IES optimization scheduling model is constructed, the refinement of the P2G two-stage operation process, and the consideration of the uncertainty of wind power and load side. Through analysis, it is found that the stepwise carbon trading mechanism is more binding on carbon emissions compared with the traditional model, which can better guide the effect of carbon emission reduction. In addition, because the uncertainty of wind generation and load side will have a great impact on the total cost of the system, improving the accuracy of wind generation prediction is important to promote the economy of system operation. On the other hand, with the continuous maturity of P2G technology and the reduction of cost, the P2G system can be applied on a large scale. In the next step, the microgrid IES can be applied to the regional power grid to realize the consumption of large-scale wind generation. The fuzzy optimal scheduling model can consider both the risks and costs of the system and provide a good reference for the subsequent study of multiple uncertainties in the process of energy scheduling.
At present, most research does not consider the demand constraints within shorter scheduling time intervals, such as on an hourly scale. In addition, method improvements based on actual data sources and method mobility analysis in different regions are lacking. These are the next research directions for this paper.
DECLARATIONS
Authors’ contributionsMajor contributions in conducting the simulation and writing the paper: Qiao J, Yin XG
Revision of the manuscript: Lu Q, Yin X
Validation of results and Manuscript revision: Liu Z, Wang Y, Zhu L
All authors read and approved the final manuscript.
Availability of data and materialsNot applicable.
Financial support and sponsorshipThis work was supported by the Fundamental Research Funds for the Central Universities, HUST: 2023JYCXJJ019, and the National Natural Science Foundation of China under Grant 51877089.
Conflicts of interestAll authors declared that there are no conflicts of interest.
Ethical approval and consent to participateNot applicable.
Consent for publicationNot applicable.
Copyright© The Author(s) 2023.
REFERENCES
1. Cheng Y, Zhang N, Zhang B, Kang C, Xi W, Feng M. Low-carbon operation of multiple energy systems based on energy-carbon integrated prices. IEEE Trans Smart Grid 2020;11:1307-18.
2. Liu J, Sun W, Harrison GP. The economic and environmental impact of power to hydrogen/power to methane facilities on hybrid power-natural gas energy systems. Int J Hydrogen Energ 2020;45:20200-9.
3. Qiao J, Yin X, Wang Y, Lu Q, Tan L, Zhu L. A stator internal short-circuit fault protection method for turbo-generator based on instantaneous power oscillation ratio. IEEE Trans Energy Convers 2023;38:1903-12.
4. Qiao J, Yin X, Wang Y, Lu Q, Tan L, Zhu L. A rotor ground fault protection method based on injection principle for variable speed pumped storage generator-motor. IEEE Trans Power Delivery 2023;38:1159-68.
5. Li Z, Yu Q, Gong W, Zhang Z. Multi-objective Optimal Scheduling of Electricity-gas-heat Energy Hub Considering Fuzzy Chance Constraint. Proceedings of the CSU-EPSA 2021;33:49-56. (in Chinese).
6. Qiao J, Yin X, Wang Y, Tan L, Lu Q. A precise stator ground fault location method for large generators based on potential analysis of slot conductors. IEEE Trans Power Delivery 2022;37:5203-13.
7. Li Y, Liu W, Zhao J, et al. Optimal dispatch of combined electricity-gas-heat energy systems with power-to-gas devices and benefit analysis of wind power accommodation. Power Syst Technol 2016;40:3680-9. (in Chinese).
8. Wei Z, Huang Y, Gao H, Yue S. Joint economic scheduling of power-to-gas and thermoelectric decoupling CHP in regional energy internet. Power Syst Technol 2018;42:3512-20. (in Chinese).
9. Gabrielli P, Gazzani M, Mazzotti M. Electrochemical conversion technologies for optimal design of decentralized multi-energy systems: modeling framework and technology assessment. Appl Energy 2018;221:557-75.
10. Schlachtberger DP, Brown T, Schäfer M, Schramm S, Greiner M. Cost optimal scenarios of a future highly renewable European electricity system: exploring the influence of weather data, cost parameters and policy constraints. Energy 2018;163:100-14.
11. Liu J, Zhou C, Gao H, Guo Y, Zhu Y. A day-ahead economic dispatch optimization model of integrated electricity-natural gas system considering hydrogen-gas energy storage system in microgrid. Power Syst Technol 2018;42:170-9.
12. Bludszuweit H, Dominguez-Navarro JA, Llombart A. Statistical analysis of wind power forecast error. IEEE Trans Power Syst 2008;23:983-91.
13. Ai X, Liu X, Sun C. A fuzzy chance constrained decision model for unit commitment of power grid containing large-scale wind farm. Power Syst Technol 2011;35:202-7. Available from: http://www.alljournals.cn/view_abstract.aspx?pcid=5B3AB970F71A803DEACDC0559115BFCF0A068CD97DD29835&cid=6CDB4E49EF88F71A&jid=F7DABF51176EF1064E985A3940C43702&aid=E1559E56DEA172DEA692DFF15C083131&yid=9377ED8094509821. [Last accessed on 27 Sep 2023].
14. Xiao D, Wang C, Zeng P, Sun W, Duan J. A survey on power system flexibility and its evaluations. Power Syst Technol 2014;38:1569-76.
15. Zhao J, Zheng T, Litvinov E. A unified framework for defining and measuring flexibility in power system. IEEE Trans Power Syst 2016;31:339-47.
16. Wang L, Liu L, Zhang K, Xiong Z, Jiang C, Guan S. A review of power system flexible ramping product and market mechanism. Power Syst Technol 2022;46:442-52.
17. Jiang T, Yuan C, Zhang R, et al. Exploiting flexibility of combined-cycle gas turbines in power system unit commitment with natural gas transmission constraints and reserve scheduling. Int J Elec Power Energy Syst 2021;125:106460.
18. Zhang L, Luo Y, Luo H, et al. Scheduling of integrated heat and power system considering multiple timescale flexibility of CHP unit based on heat characteristic of DHS. Proceedings of the CSEE 2018;38:985-98.
19. Ma Z, Jia Y, Han X, Kang L, Ren H. Two-layer dispatch model of integrated energy system considering dynamic time-interval. Power Syst Technol 2022;46:1721-30. (in Chinese).
20. Tan Q, Ding Y. Optimal energy-saving dispatching model for thermal power considering carbon trading and its coping mode. Electric Power Automation Equipment 2018;38:175-81. (in Chinese).
21. Zhang X, Yan K, Lu Z, Zhong J. Scenario probability based multi-objective optimized low-carbon economic dispatching for power grid integrated with wind farms. Power Syst Technol 2014;38:1835-41. (in Chinese).
22. Zhou L, Zhou Y. Study on thermo-electric-hydrogen conversion mechanisms and synergistic operation on hydrogen fuel cell and electrochemical battery in energy flexible buildings. Energy Convers Manag 2023;277:116610.
23. Yang L, Sun Q, Zhang N, Li Y. Indirect multi-energy transactions of energy internet with deep reinforcement learning approach. IEEE Trans Power Syst 2022;37:4067-77.
24. Wang X, Liu R, Wang X, Hou Y, Bouffard F. A data-driven uncertainty quantification method for stochastic economic dispatch. IEEE Trans Power Syst 2022;37:812-5.
25. Li Y, Gao DW, Gao W, Zhang H, Zhou J. Double-mode energy management for multi-energy system via distributed dynamic event-triggered newton-raphson algorithm. IEEE Trans Smart Grid 2020;11:5339-56.
26. Wei Z, Zhang S, Sun G, Xu X, Chen S, Chen S. Carbon trading based low-carbon economic operation for integrated electricity and natural gas energy system. Automation Electr Power Syst 2016;40:9-16.
27. Cui Y, Yan S, Zhong W, Wang Z, Zhang P, Zhao Y. Optimal thermoelectric dispatching of regional integrated energy system with power-to-gas. Power Syst Technol 2020;44:4254-64. (in Chinese).
28. Jiang C, Ai X. Integrated energy system operation optimization model considering uncertainty of multi-energy coupling units. Power Syst Technol 2019;43:2843-54. (in Chinese).
Cite This Article
Export citation file: BibTeX | RIS
OAE Style
Qiao J, Yin XG, Lu Q, Liu Z, Wang Y, Zhu L, Yin X. Optimal scheduling of electricity-gas-heat-hydrogen integrated energy system considering carbon transaction cost. J Smart Environ Green Comput 2023;3:106-21. http://dx.doi.org/10.20517/jsegc.2023.12
AMA Style
Qiao J, Yin XG, Lu Q, Liu Z, Wang Y, Zhu L, Yin X. Optimal scheduling of electricity-gas-heat-hydrogen integrated energy system considering carbon transaction cost. Journal of Smart Environments and Green Computing. 2023; 3(4): 106-21. http://dx.doi.org/10.20517/jsegc.2023.12
Chicago/Turabian Style
Qiao, Jian, Xiang-Gen Yin, Qinghui Lu, Zhichang Liu, Yikai Wang, Lingjin Zhu, Xin Yin. 2023. "Optimal scheduling of electricity-gas-heat-hydrogen integrated energy system considering carbon transaction cost" Journal of Smart Environments and Green Computing. 3, no.4: 106-21. http://dx.doi.org/10.20517/jsegc.2023.12
ACS Style
Qiao, J.; Yin X.G.; Lu Q.; Liu Z.; Wang Y.; Zhu L.; Yin X. Optimal scheduling of electricity-gas-heat-hydrogen integrated energy system considering carbon transaction cost. . J. Smart. Environ. Green. Comput. 2023, 3, 106-21. http://dx.doi.org/10.20517/jsegc.2023.12
About This Article
Copyright
Data & Comments
Data
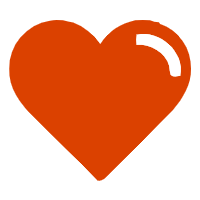

Comments
Comments must be written in English. Spam, offensive content, impersonation, and private information will not be permitted. If any comment is reported and identified as inappropriate content by OAE staff, the comment will be removed without notice. If you have any queries or need any help, please contact us at support@oaepublish.com.